impact
- 45% reduction in the current cost of maintenance for railway crossings.
- 30% reduction in urgent replacements of railway crossings.
Developed AI Technologies
- Advanced self-learning ML technology for precise detection & identification of railway crossings failures in a multi-sensor environment.
- Scalable & flexible decentralized architecture with rapid deployment, optimizing data processing efficiency.
- Real-time updates for proactive maintenance strategies, leveraging Edge-based machine learning algorithms.
- Comprehensive data collection through Federated IoT monitoring, streamlining data acquisition for ML-based insights.
- Proven results with reduced maintenance costs, improved safety, and optimized training needs using advanced ML techniques.
- Innovative IIoT solution with machine learning at its core for data-driven product innovation and performance measurement.
Challenges
Challenges in railway crossing maintenance are supported by figures from market data and references. For instance, studies indicate that regular inspections are necessary to prevent accidents and ensure safety, with some railway operators conducting inspections as frequently as multiple times per day (Transportation Research Board, 2017). Effective stakeholder coordination is crucial, as railway crossings often involve coordination among multiple entities, including railway operators, government agencies, and local communities (World Bank, 2018).
Infrastructure upgrades, such as improving crossing surfaces and installing warning devices, can be costly, with estimates indicating that the average cost of upgrading a railway crossing can range from tens of thousands to millions of dollars (Market Research Future, 2019).
Regulatory compliance is also a significant challenge, with railway crossings subject to various federal, state, and local regulations related to safety, accessibility, and environmental impact (American Association of State Highway and Transportation Officials, 2020). Environmental exposure, such as extreme weather conditions, can cause wear and tear on railway crossings, leading to increased maintenance needs (Transportation Research Board, 2017). The adoption of advanced technologies for predictive maintenance and automation is gaining traction, with the global railway predictive maintenance market projected to reach USD 6.5 billion by 2027 (Market Research Future, 2019). However, implementation and integration challenges, such as data privacy concerns, interoperability issues, and system complexity, can pose hurdles (Transportation Research Board, 2017). Overcoming these challenges is imperative for ensuring safe and reliable railway crossing operations, reducing maintenance costs, and improving overall efficiency (World Bank, 2018).
results
A cutting-edge solution for railway crossing maintenance is being developed, utilizing an innovative automatic detection and identification system. This system is based on self-learning algorithms that operate within decentralized architectures and leverage real-time information.
It enables continuous evaluation for predictive maintenance of railway crossings, incorporating ultrasonic inspection and leveraging federated IoT monitoring and machine learning techniques in dynamic scenarios. This advanced system has the potential to revolutionize railway crossing maintenance by providing real-time insights, facilitating proactive maintenance strategies, and improving overall operational efficiency.
With its state-of-the-art approach, this solution aims to enhance the safety, reliability, and performance of railway crossings, ensuring smooth and uninterrupted operations.
This ambitious project has been successfully carried out in the city of Malaga, with a duration of 18 months and a budget investment of €1,410,500. AINGURA has been part of a consortium (with a participation higher than 35%), led by AMURRIO Ferrocarril y Sistemas and with the participation of Tecnalia and Dasel Sistemas
This initiative is co-financed by the European Regional Development Fund (FEDER) under the framework of the Multi-Regional Operational Program of Spain 2014-2020.
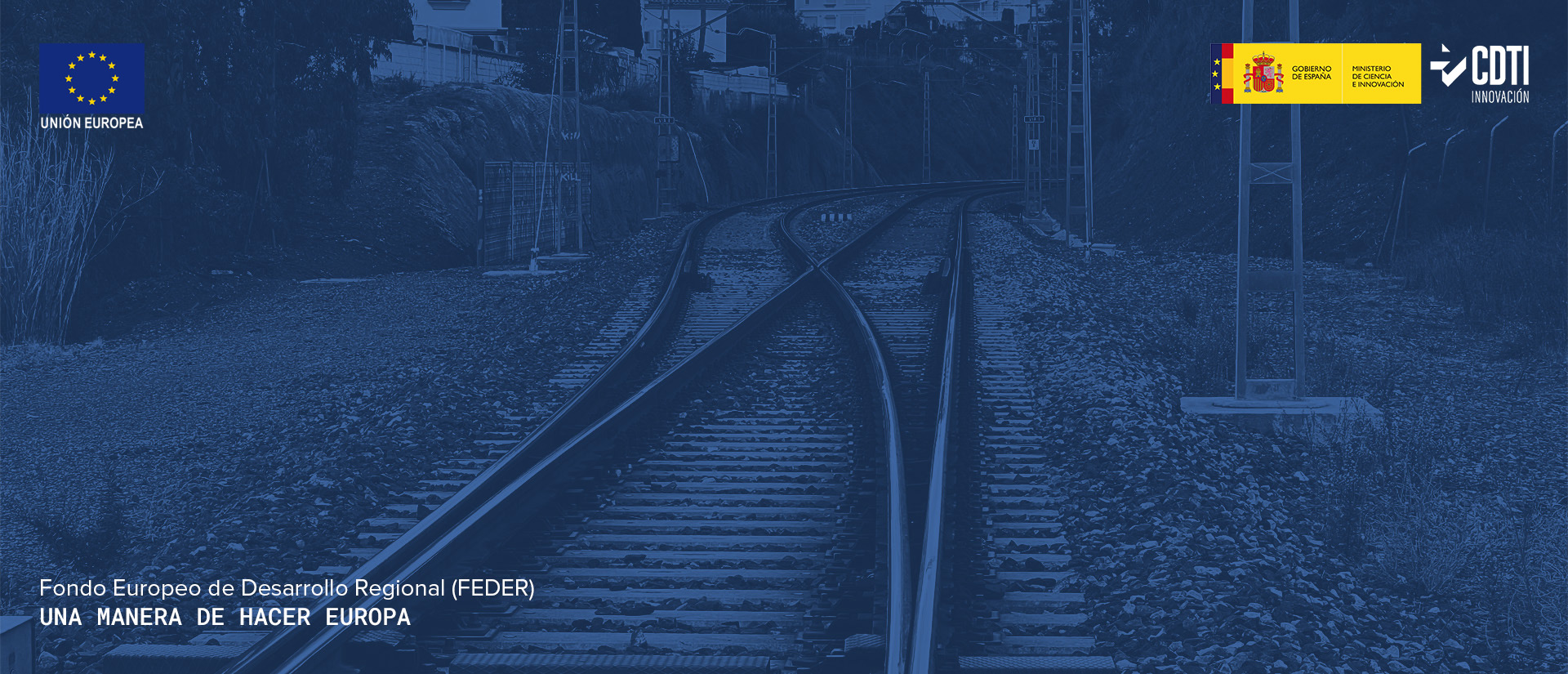