impact
- Cluster 1 has been found as an anomalous consumption pattern, which represents 14% of the global energy consumption of the cooling system.
Developed AI Technologies
- Machine Learning.
- Pattern analysis, prediction and intelligent classification.
Challenges
- Improvement of energy efficiency of cooling systems in the food sector.
- To search for consumption patterns that are deviated from the normal operating pattern of the cooling system.
results
- Dataset size: 10GB
- Number of variables: 45
- Sampling time: 15s
- Data from the SCADA system of the production plant have been used to analyze the consumption of the cooling system
- The cooling system already had a large amount of investment in elements and strategies for energy efficiency. However, due to the high energy bill, there was interest in seeing the possibility of finding anomalous patterns of operation that may be influencing in the energy consumption.
- An exploratory clustering algorithm based on Gaussian mixture models was used to:
- Find the energy consumption pattern of the system.
- Compare energy consumption against normal consumption pattern to detect anomalies.
- Additionally, multidimensional scaling techniques have been used to facilitate the visualization of variables.
- A consumption pattern has been developed like the one shown in Figure 4, which shows normal consumption (Cluster 0 and 2) and abnormal consumption (Cluster 1)
- The events collected in this Cluster have been isolated and delivered to the client for analysis, in order to determine what has caused this type of deviation.
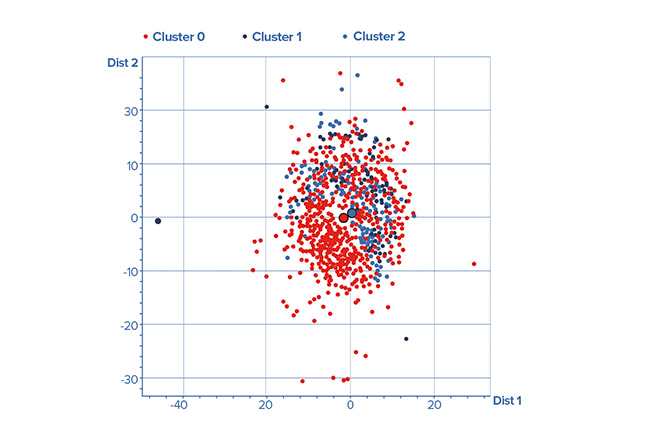
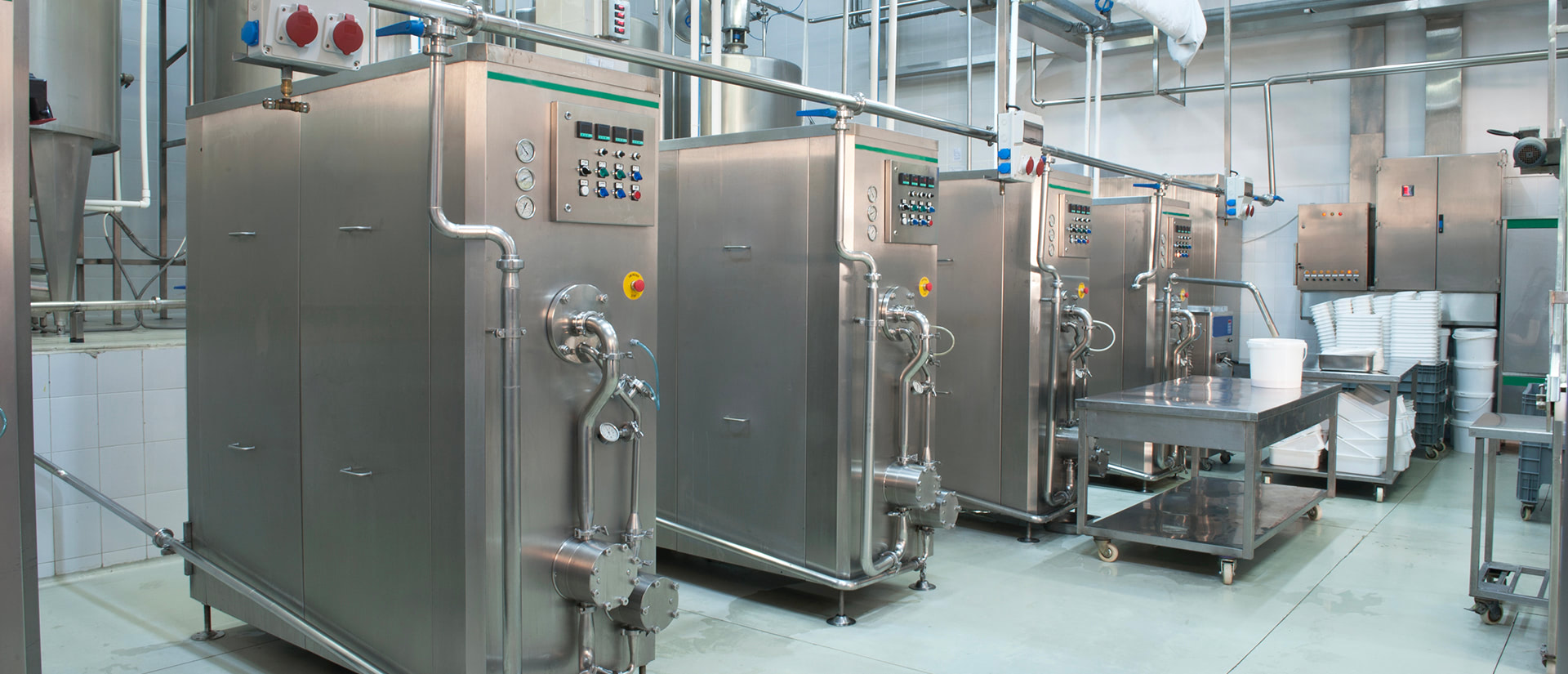