Participants
Etxetar
Universidad Politécnica de Madrid
impact
- Detect deviations in the process parameters below 15% of the cycle time, commonly invisible to the deployment technicians.
- Increased productivity by an average of 6 hours a day.
Developed AI Technologies
- Data intelligence.
- Machine Learning.
- Pattern analysis, prediction and intelligent classification.
Challenges
- To increase in machine availability in high production systems in order to maintain or exceed 95%.
- To search for inefficiencies in the operating pattern that represent losses of time and prevent compliance with line cycle times.
- Reduction of machine deployment times by detecting imperceptible deviations.
results
- Dataset size: 12GB
- Number of variables: 22
- Tiempo de muestreo: 100ms
- Development of an algorithm for the detection of deviations based on a hybrid system between Kernel Density Estimation (KDE) and Hidden Markov Models (HMM) for training and detection of deviations based on machine data acquired at 100 ms.
- Offline data analysis system that has allowed to find deviations below 2 seconds, as shown in graphics bellow.
- The inefficiency detected is related to a CNC code line that unexpectedly moved the drilling references in the B axis. This error would have been practically impossible to detect with the naked eye.
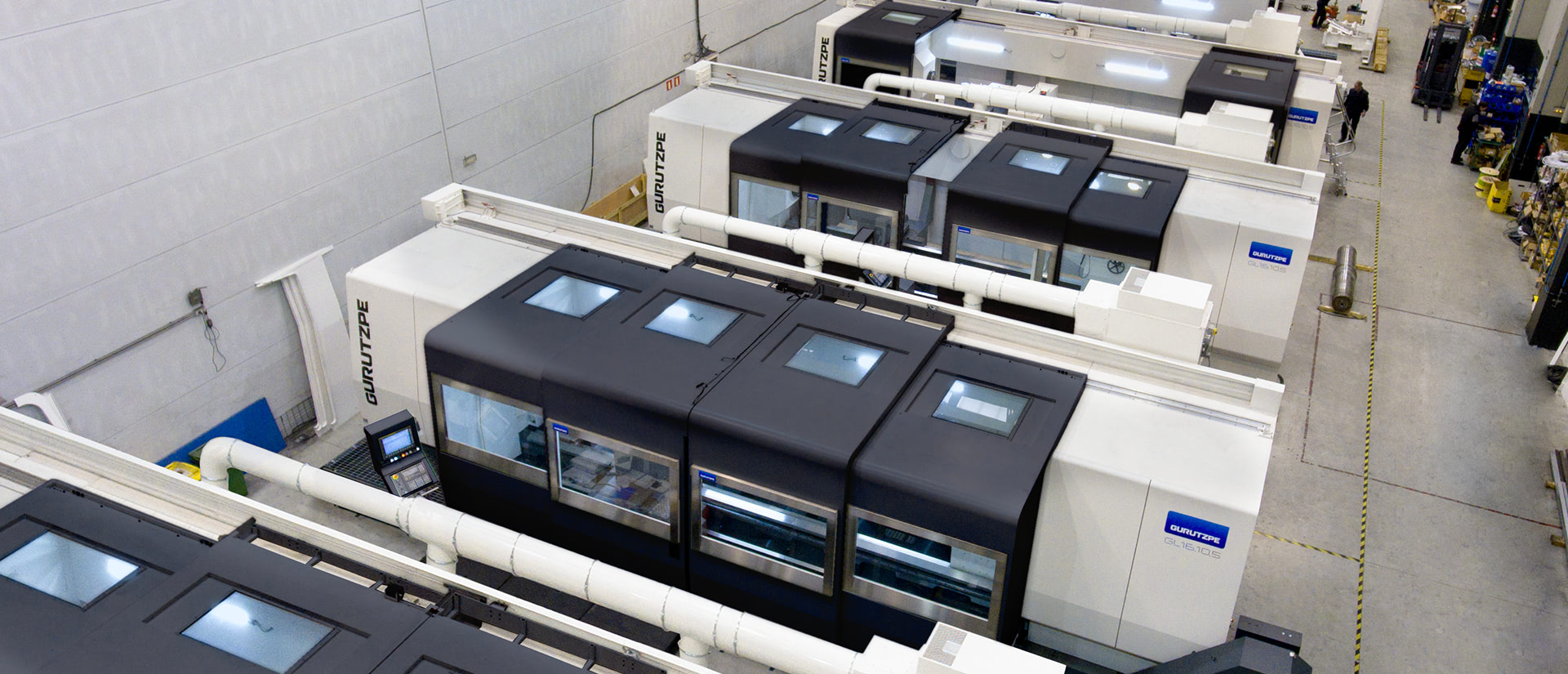