Participants
Etxetar
Universidad Politécnica de Madrid
impact
- 8% improvement in machine availability.
- Improved machine design by detecting the part of the machine that is most sensitive to thermal changes.
Developed AI Technologies
- Data intelligence.
- Smart sensors.
- Intelligent automation.
- Machine learning.
- Pattern analysis, prediction and intelligent classification.
Challenges
- To increase in machine availability in through high temperature gradients in production facilities. Due to these gradients, the machine/manufacturing line had to be idle around 2 hours.
- Support to the PLC/CNC compensation system to withstand extreme temperature changes.
- Improvement of the machine design according to the study of the sensitivity of different parts of the machine to extreme changes in temperature in a real productive environment.
- To complement analyzes done with finite elements during the machine design phase.
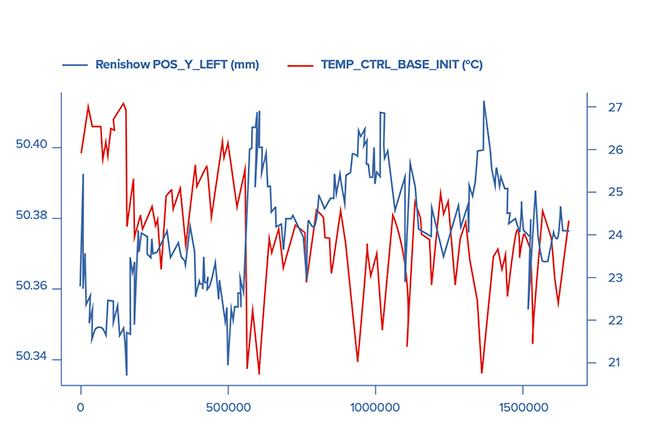
results
- Dataset size: 2GB
- Number of variables: 15
- Sampling time: 100ms
- Development of the first unattended system for the acquisition and pre-processing of machine data and interaction with the PLC.
- A predictive system for tool tip positioning has been developed.
- Development of an off-line variable selection model.
- Development of a multi-output regression model for continuous variables of temperature and flow.
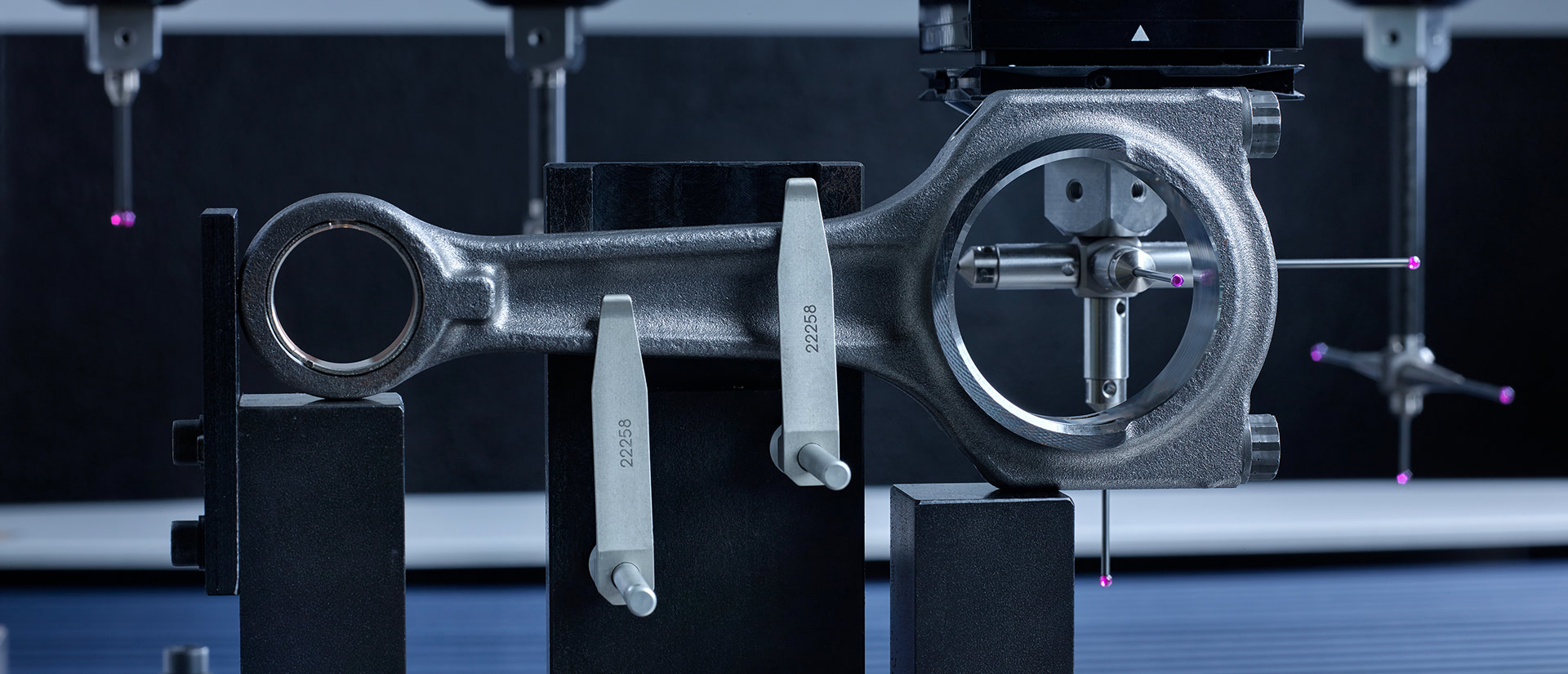