Participants
Etxetar
Universidad Politécnica de Madrid
impact
- Machine monitoring using machine learning techniques appropriate for application in dynamic environments, where failures are not very common and are related to degradation.
- Monitor in real time the status of the bearings with respect to the generated reference.
Developed AI Technologies
- Data intelligence.
- Machines/people interfaces.
- Smart sensors.
- Machine learning.
- Pattern analysis, prediction and intelligent classification.
Challenges
- To develop machine monitoring system that allows failure detection in critical elements, in this case, the spindle header.
- To develop first steps for the detection of anomalies in highly dynamic processes.
results
- Dataset size: 1GB / day
- Number of variables: 10 - 110
- Sampling time: 50 - 100ms
- It has been possible to develop data acquisition systems that ensure their quality by extracting them from production systems such as machine tools.
- Once this quality was assured, the first machine patterns were developed.
- These patterns have been examined and compared as a reference to detect possible deviations in relation to the reference.
- This system allows monitoring the state of the front bearing of the spindle head. Its failure can cause the machine and production line to stop.
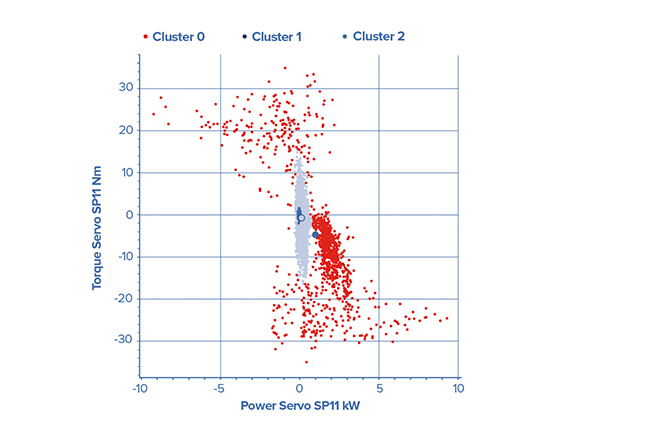
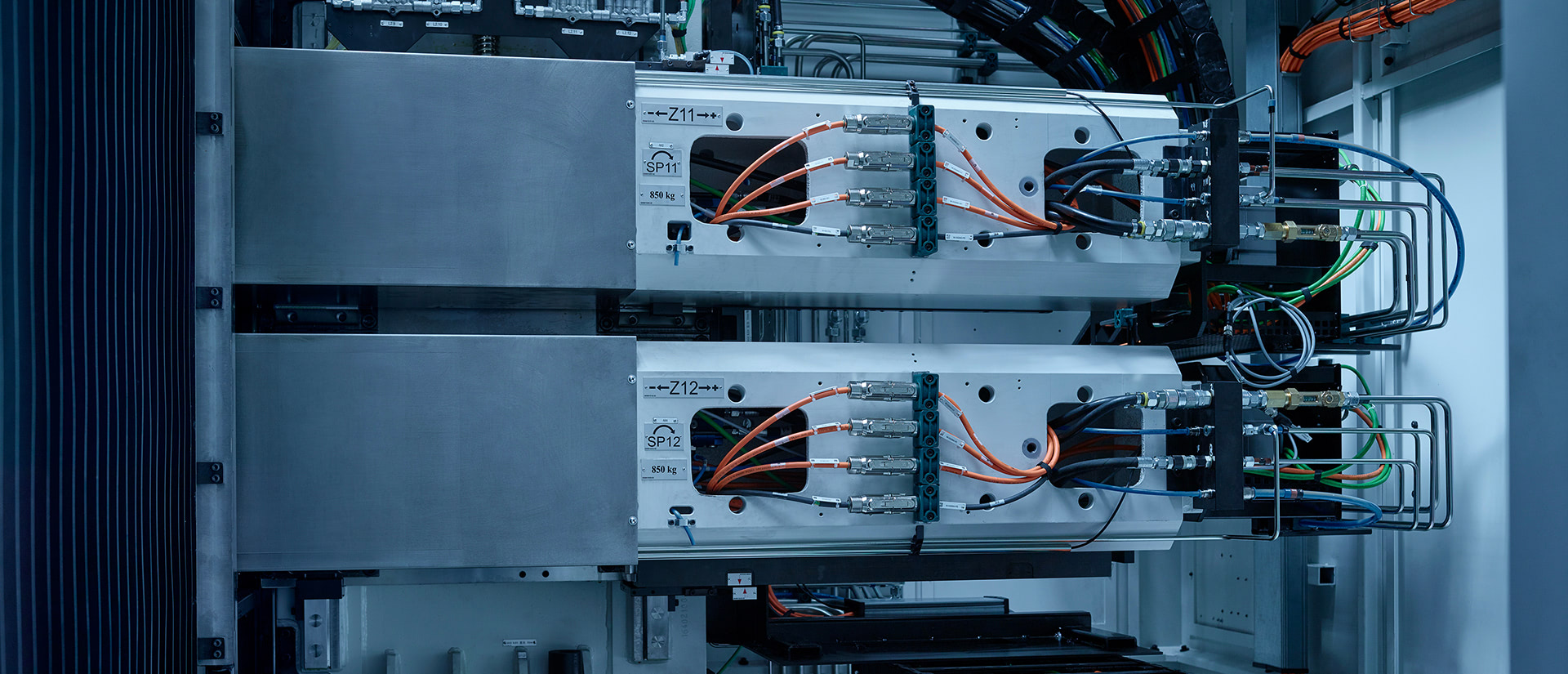
Publications
Author: J. Diaz-Rozo, C. Bielza, y P. Larrañaga
Title: Machine learning-based CPS for clustering high throughput machining cycle conditions
Publication: Procedia Manufacturing, vol. 10, pp. 997-1008
Date: January 2017