impact
- Potential Improvement in energy efficiency between 7% and 10% depending on equipment.
- Potential improvement in energy consumption by 15% approx. in support systems such as cooling.
Developed AI Technologies
Pattern analysis, prediction and intelligent classification
Challenges
- To explore production system consumption pattern to detect hidden electric energy consumers that are large enough to impact in the overall energy consumption.
- To provide an advanced level of energy consumption analysis after applying traditional efficiency techniques.
- To detect outlier situations where energy consumption is deviated from normal consumption to include them in the next version of company’s environmental and energy consumption policies.
results
- Dataset size: 500 MB.
- Number of variables: 7.
- Sampling time: 1s.
- Exploratory clustering algorithms (Gaussian mixtures, k- means, agglomerates) have been applied to search for atypical consumers or energy consumption deviations.
- Multidimensional scaling techniques have been used to visually analyze these patterns.
Figure 17. Consumption pattern of the food coating line.
- A temporal analysis has also been carried out on the consumption pattern. A deviation has been detected related to a fire in the capacitor bank a month later. This is shown in Figure 18.
![]() |
![]() |
![]() |
Figure 18. Temporal analysis of the consumption pattern.
- Cluster 0 and 1 show the change in the behavior of the system from the energy point of view at the beginning of September. Cluster 1 contains all the data from that change until the fire in late October.
- Cluster 2 also shows other random consumption patterns that imply an increase in the average consumption of the line, which should be studied.
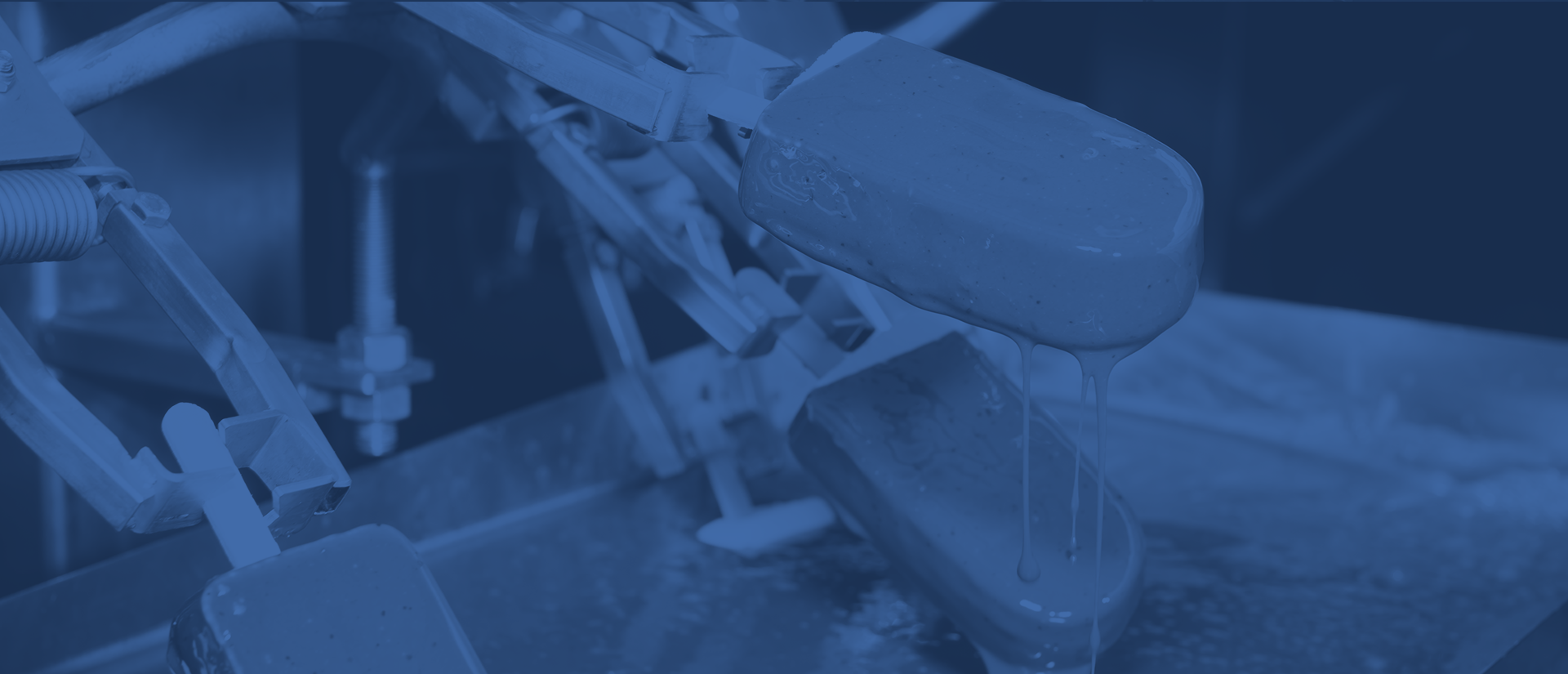